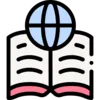
EduPEF: Educational Personalized Feedback for Mathematics
Try our feedback generator
This tool is designed to leverage Large Language Models (LLMs) to generate personalized feedback for mathematical multiple-choice questions, enhancing the learning experience through adaptive educational technology. With the human-like text generation capabilities of LLMs, this tool aims to offer tailored feedback that aligns with the specific knowledge state and learning preferences of students—areas that have been relatively underexplored in prior research focusing mainly on generic instructions and exercises. The tool compares stock, automatic, and adaptive feedback types. Stock feedback is human-generated and provides a clear strategy for solving problems, while automatic and adaptive feedback are generated by LLMs. Automatic feedback focuses solely on question-specific details, whereas adaptive feedback integrates data on student profiles to personalize the response further.
The effectiveness of these feedback types is assessed in guiding students from their current understanding to a desired knowledge state. To ensure quality and reliability, feedback is evaluated by human experts who consider student profile details and feedback alternatives, using 5-point Likert scale ratings to assess aspects such as content, tone, and engagement. The findings indicate that LLM-generated feedback, especially when incorporating student profiles, shows potential for medium- and low-performing students by better addressing individual needs compared to standard stock feedback. Although the integration of learning style model attributes did not consistently show significant quality improvements, LLM-generated feedback proved impactful in enhancing content quality and engagement. However, tone consistency among different types of feedback remains a subjective measure among expert raters.
Ultimately, this tool aims to provide a foundation for developing more adaptive and scalable feedback systems within Intelligent Tutoring Systems (ITS). It is designed to support different student profiles, improve learning outcomes, and offer practical guidelines for integrating LLMs into educational technology for personalized learning experiences.